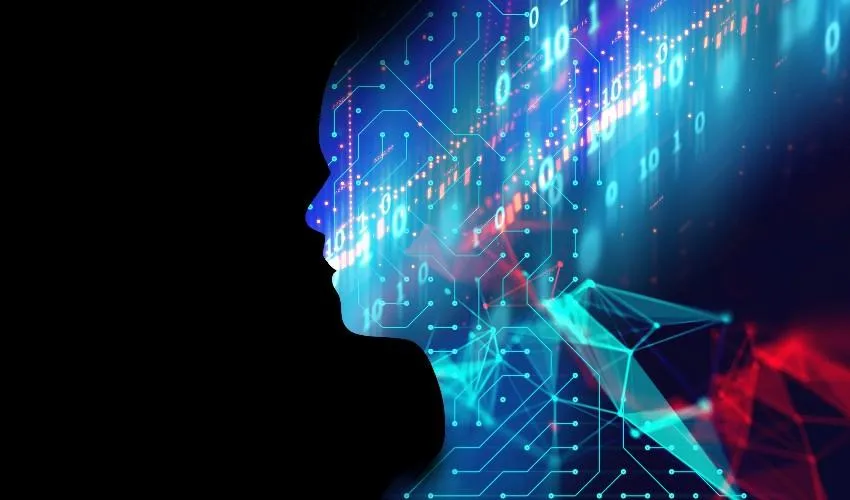
Sviluppi del deep learning per scienziati sociali
Importanti accademici di tutto il mondo presentano la loro ricerca più avanzata ogni anno in Bocconi nel corso di seminari aperti a professori e studenti. Per rendere tali risultati accessibili a un pubblico più ampio, Bocconi Knowledge pubblica le sintesi (in inglese) dei seminari scientifici e di policy organizzati dal centro di ricerca IGIER, a cura degli studenti dell'IGIER-BIDSA Visiting Students Initiative.
Suppose that you wanted to understand how state control affects firm productivity and you have an archive with many books containing highly detailed information about state-controlled firms in Japan. Hiring people to collect the data, spanning thousands of pages, is way too costly and impractical. Using standard available Optical Character Recognition (OCR) tools on the scanned pages is also infeasible, for the output would be too imprecise and hence hard to use. Must you abandon the project? The question is very relevant in social sciences, in which large amounts of potentially useful data is stored in extensive archives that cannot be systematically collected and analysed using standard techniques. To unleash this information, we need technological innovation!
On the 16th March installment of the 2020-2021 IGIER Seminar Series, Professor Melissa Dell, from Harvard University, explained how Artificial Intelligence techniques, in particular Deep Learning, can be a crucial asset in this endeavor, and talked about her work geared at making such tools accessible and available for social science research.
Deep learning makes use of complex architectures called neural networks, that were initially developed to mimick the way a brain actually learns. Compared to traditional methods, in deep learning the computer learns the decision rules on its own, which makes the results more robust to noisy data and more easily generalizable.
Professor Dell argued that in social science these methods can be useful assets to work with traditional data sources, but especially to unlock the potential coming from sources that were previously deemed unfeasible to analyse.
Imagine, for instance, that you wanted to study the evolution of political ideologies, using information contained in historical newspapers. First of all, conventional OCR methods would be highly ineffective: they often fail when faced with complex or unusual page layouts and they may extract text that is incomplete, at best, and nonsensical, at worst (by mixing different sections and columns together). Such inaccurate retrieval would then only allow for simple queries, such as keyword search, and would make it impossible to carry out more sophisticated language and topic modelling tasks.
Dell argued, instead, that customized OCR, using neural networks, can be fundamental to be able to accurately extract text from scanned pages. Once we have our full text in machine-readable form, state-of-the-art and fully open-source models (like BERT and RoBERTa, by Google and Facebook) can achieve incredibly accurate performance in natural language understanding.
Another domain of interest is the case, discussed at the outset, of historical disaggregated data. For economists, it is a widely accepted reality that many research questions can only be understood, with sufficient granularity, by using microeconomic data. It is however hard to find disaggregated data, covering long enough periods of time, in digitized format.
Dell showed that in this case one can use Generative Adversarial Networks (GANs) to "clean-up" noisy scans, warped or worn out by time. Then, to extract different elements, such as from accounting records, one can rely on object detection models based on Convolutional Neural Networks (CNN). Lastly, it is possible to train customized OCR engines, for instance using Encoder-Decoder architectures, when dealing with unusual fonts that are not recognized by commercial OCR tools.
Deep learning can thus be a valuable addition to every step of the curation pipeline: from the layout analysis and pre-processing to the actual task of natural language processing. And, although these problems seem to be entirely different from each other, the techniques actually used to tackle them are remarkably similar.
These methods have large advantages not only relative to existing off-the-shelf tools, but also to manual data curation. Besides its large cost, in fact, manual data entry is also prone to errors, since it often relies on commercial OCR software as a first pass. It may even be simply unfeasible for very large datasets, as is the case for the incredibly valuable disaggregated microeconomic data. Deep learning can of course also be costly, but after the initial investment in computing resources and human capital, it scales very well. Automated data curation can thus help democratize the access to data and empirical research, and it can allow social scientists to raise new questions and study new contexts, such as those of lower-income countries for which data is available in physical form but is seldom digitized.
In order to make these tools more accessible to social scientists, unaccustomed with the field of computer science, Professor Dell and her group have been working in two directions. First, by providing open-source toolkits for researchers, to carry out layout detection, OCR and NLP tasks, in a way that is as user friendly as possible for any Python user. Then, she has been working on sharing notes and resources to allow for a gentler introduction to the field of neural networks, which is extremely vast and fast-paced.
She concluded by restating how deep learning can be key in unlocking massive information currently trapped in text and image data and encouraging researchers to become familiar with such methods and how they can be applied to social science.