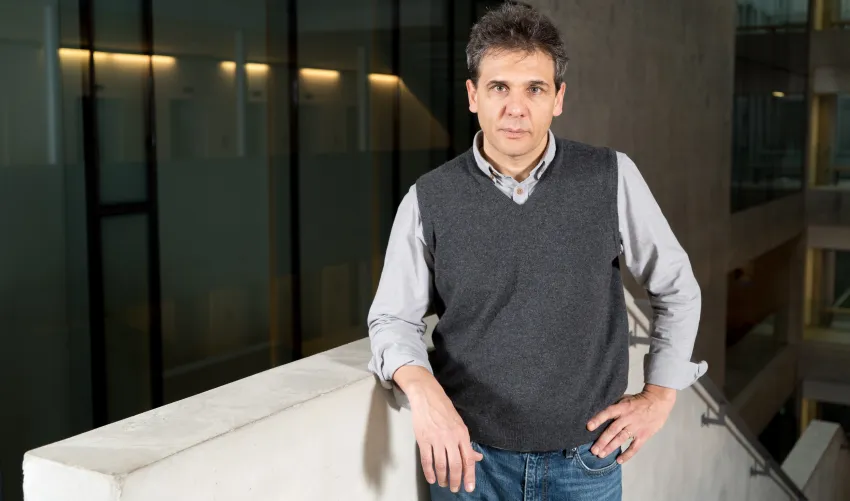
A Multidisciplinary Approach is Key to Unleashing AI
For most of us, 2012 was simply the year when the world didn't end, despite the Maya prophecy. Some can also remember the first observation of the Higgs boson at Geneva's CERN or Felix Baumgarten, the Austrian who parachuted in free fall from 39,000 meters, becoming the first man to overcome the speed of sound. On the other hand, there are only a few traces of the turning point that started the revolution, at first silent and then increasingly thunderous, of Artificial Intelligence (AI). «In 2012», explains Riccardo Zecchina, a theoretical physicist and Director of Bocconi's new Bachelor of Science in Mathematical and Computing Sciences for Artificial Intelligence, «a neural network drastically improved the accuracy with which a machine is able to recognize an object within an image dense with objects, in ImageNet's Large Scale Visual Recognition Challenge».
It sounds like Where's Waldo? Is it really such a remarkable achievement?
Absolutely. To put it simply, there are two approaches in Artificial Intelligence. On the one hand, there is the classic one, now called Artificial General Intelligence, which dates back to the '60s and is based on logics and deductive methods. On the other hand, there is machine learning, or automatic learning, which uses methods that start from data. In 2012, the algorithmic results on ImageNet seemed to have reached a ceiling, and in the recognition exercise the error rate settled at 26%, compared to the average 5% of humans: Machine learning (in particular the so-called Deep Learning that makes use of artificial neural networks) or Data-Driven AI, had entered the spotlight. Using the new approach, all of a sudden (even if laying out the neural network had been long and demanding) the error rate dropped to 16%. A totally unforeseen fact. We're really talking about yesterday, but that's when the world realized the potential of artificial intelligence. Myriad applications followed and, in the meantime, the error rate in that particular exercise has fallen to 2%. Machines are now more accurate than humans in some specific visual recognition exercises, and can perform them at an incomparably faster rate.
But what can machine learning do?
Machine learning includes many different methods, used in different situations. All of them extract information from data. In the case of ImageNet, and more generally in the most recent advances, the method that is producing the most innovative and surprising results is Deep Learning, which is based on models of neural networks that recall, in a very stylized way, the workings of the human visual system. A network of nodes capable of processing information is created, arranged in numerous layers and with numerous links between nodes and it is «trained» with an iterative learning process: if I submit the image of a cat and the system does not recognize it, I modify the conformation of the network until it does so and then I submit another image, and so on. It is a very different logic from that of traditional artificial intelligence, and in fact it has developed in laboratories isolated from the mainstream and involving different disciplines, because it poses issues of a multidisciplinary nature, regarding conceptualization, optimization and modeling.
It is clear to everyone how AI impacts on technology, but why does the revolution also involve social sciences?
Artificial intelligence is changing the way both natural and social sciences work, when data must be processed. In the meantime, it has made possible operations that we would not even have dreamed of before, such as speech recognition, an acceptable machine translation, climate change modelling or programs that describe the content of an image to the blind. In other cases, such as that of search engines on the Internet or in the Internet of Things, artificial intelligence has drastically improved the results. In management, companies ask to use data to improve their processes. In healthcare, machine learning enables the implementation of large-scale automated diagnoses and personalized medicine. Finance also relies on quantitative methods. In general, machine learning can become an extremely useful tool for monitoring and optimizing all development processes that need to become climate-change proof.
The very concept of Artificial Intelligence also raises some concerns. Is there any reason for this?
In order not to move from science to fiction, one thing must be clear: Artificial Intelligence does not replace our ability to make decisions. It extracts information, it builds models, it is an extra support, like having a co-processor, but we must continue to take decisions. What we expect in the next few years is not the singularity - Artificial Intelligence taking power - but a series of challenging decisions that establish if we want to modify some social and political systems in order to be able to exploit the potential of the new tools. Increasingly accurate mass screening, for example, will only be possible if the healthcare systems are willing to collect and make available large amounts of standardized data. The real challenge is to convince the actors to cooperate.
And what about the scientific challenges?
First of all, we must arrive at a rigorous understanding of how the most modern methods, such as machine learning, work. Applications are anticipating theoretical understanding and there is a lot of work to be done. Then, there are the open problems. The first is the transition from supervised to unsupervised recognition. In the case of the Large Scale Visual Recognition Challenge, recognition is supervised because the objects have been classified by humans before asking the machine for recognition, but the vast majority of data is not supervised. Self-supervised learning is reached when machines can infer a model from data, act according to the model and analyze the responses of the environment to their actions, modifying the model if these do not match expectations. The second problem is the Holy Grail of machine learning research: teaching machines to infer causal patterns from data. In general, machine learning is being used in all scientific disciplines, including social ones. Its applications are broad-spectrum and I believe that they will contribute significantly to scientific progress in the coming years. The development of AI and its applications will require unprecedented multidisciplinary collaboration in science, at 360 degrees.
Read more about this topic:
Training the Creators of Future