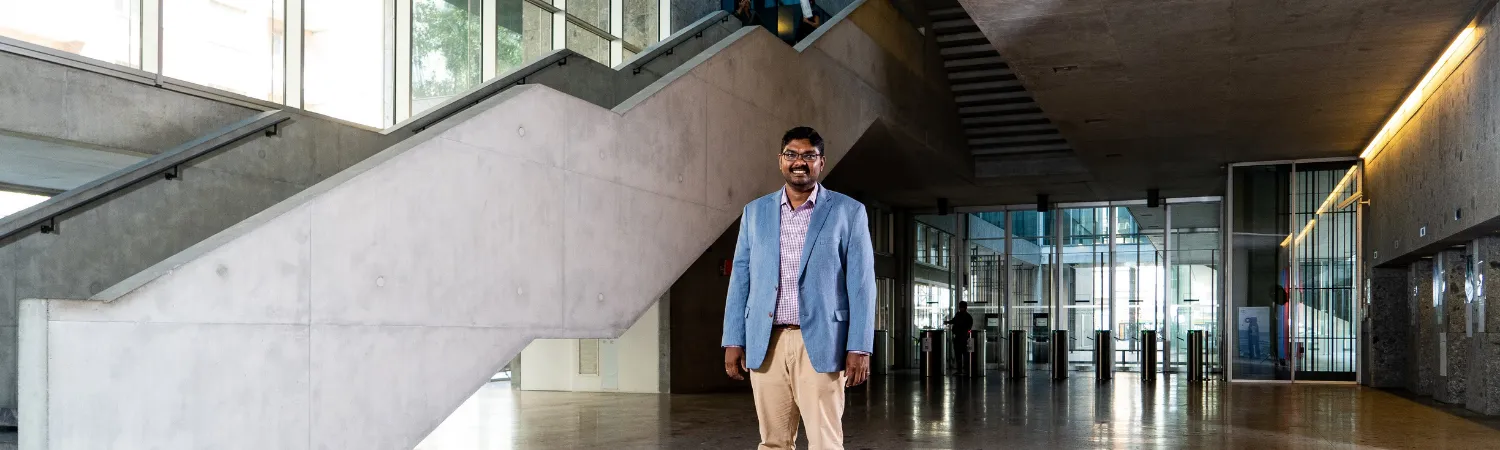
Lovely and Likely, But Maybe Wrong
Finding a convincing explanation of a complex issue is no easy task. The decision about what qualifies as the "best" solution is inevitably subject to biases and approximations. Coupling statistics with historical methods, however, makes the decision process more rigorous and less error-prone, as Sandeep D. Pillai of Bocconi's Department of Management and Technology argues in his recent paper "Lovely and likely: Using historical methods to improve inference to the best explanation in strategy", written with Brent Goldfarb and David Kirsch (both of the University of Maryland in the United States) and published in the Strategic Management Journal.
A lot of business literature is, ostensibly, about finding solutions to specific problems. These solutions are ideally broad enough to underpin a theory, or at least they are judged to be applicable to a range of cases. Yet, problems can only rarely be solved by addressing a single causal factor. Managerial issues, which apply to complex organizations like firms which operate in complex environments like markets, are bound to be influenced by a large number of interdependent factors which are hard or impossible to tell apart.
Amid such uncertainty, we are unlikely to ever discover the "true" solution to a problem. The most we can hope to achieve is the best solution, or explanation, among many. But what makes a solution appear better than another? Sandeep Pillai and his colleagues argue that the "inference to the best explanation", or IBE for short, is the process at play in these cases. Thus, explanations that are lovely, in the sense that they are useful, general, and provide meaning, and likely, in the sense that they are close to the truth, are generally adopted as the best possible. Of course, not all lovely explanations are likely as well, and vice versa. Besides, IBE will only be reliable if the true theory is among the theories being considered. But there is no reason to believe that this is the case, nor that our "best" explanation is true. This conundrum is known as the "bad lot problem", because when the truest explanation escapes us we will end up picking the best of a bad lot.
And since most phenomena in business strategy are ambiguous, inference to the best explanation often becomes an exercise in inference to a preferred explanation, and preferences are bound to be influenced by several different biases. This is not inherently a bad thing, because problems need to be addressed and deciding where to start means to go for an acceptable solution rather than finding the absolute truth. But it is important to be aware that such biases exist in order to limit their negative consequences.
Pillai and his co-authors argue that addressing strategy issues by adopting a statistical method only (that is, some kind of data analysis) is common, but may lead to falling into the bad lot problem. They suggest that "historical" methods can improve data observation and consequently enable better hypothesis generation. The term "historical methods" includes three main concepts: hermeneutics (understanding who the decision-makers are and their perspectives), contextualization (understanding decision-makers' situations and how they relate to each other), and source criticism (understanding the weight to attach to any specific record of the past).
"We use recent work in the philosophy of science to consider how we arrive at best explanations that are useful, general, provide meaning, and, at the same time, are close to the truth. Interpreting observational results requires an understanding of the context that statistical analysis alone cannot provide," says Sandeep Pillai. "Methodological tools from the field of history can improve the process of determining the best explanation by helping scholars generate new candidate explanations and systematically judge and privilege explanations. The use of historical records to apply an IBE methodology clarifies the judgments that we are making at multiple levels and helps researchers and readers evaluate these judgments as we decide what needs to be explained and what we can put aside as unhelpful."