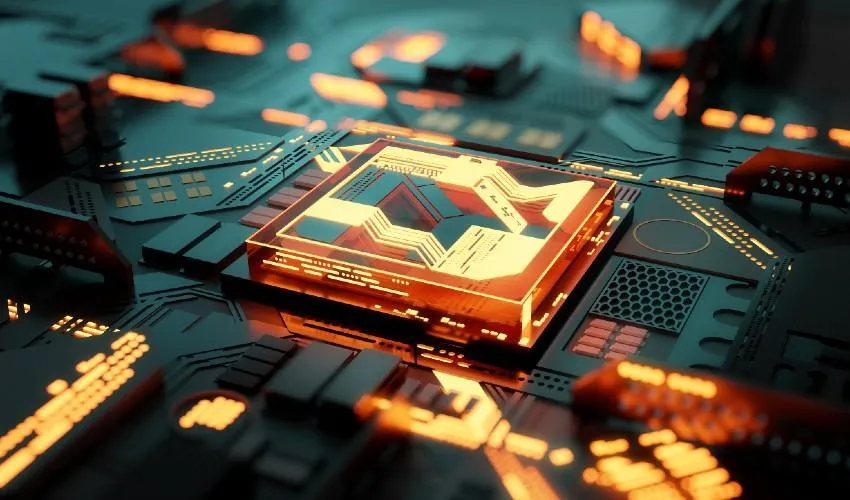
When Machines Learn Prejudices
Three researchers from Bocconi Department of Computing Sciences have demonstrated the existence of a strong bias that penalizes the LGBTQIA+ community in the world's most widely used and most powerful language model (BERT), used by the scientific community to develop countless language-related machine learning tools.
When asked to complete a neutral sentence, the BERT language model most often completes it with hurtful words if the subject is a woman rather than a man, and even more so (up to 87% of cases for terms related to certain queer identities) if the subject is LGBTQIA+.
Between 2018 and 2019, the world of Natural Language Processing (NLP) was transformed by Google's development of a new language model, BERT. Language models are used by machines to understand natural language like humans do, and BERT has achieved great results from the outset. It is precisely thanks to BERT that Google is able to infer from the context what we mean by a certain word. When we type in "spring" for example, Google comes up with images of both metal coils and flowering landscapes, but if we type in "bed spring" it shows us only metal coils and if we type in "spring nature" only landscapes.
One of the methods used to train language models is "masked language modeling": a sentence with a missing term is fed into the system and the model is asked to enter the most likely term, repeating the exercise until predictions are accurate.
Debora Nozza, Federico Bianchi and Dirk Hovy of Bocconi's Department of Computing Sciences asked BERT to carry out a similar exercise (complete a few sentences, written in six different languages) to develop a measure of the probability of returns with hurtful language (HONEST - Measuring Hurtful Sentence Completion in Language Models) and test whether there is a bias that penalizes women or the LGBTQIA+ community.
"We have observed a disturbing percentage of bias," Nozza says. 4% of male-subject sentences and 9% of female-subject sentences are completed with expressions referring to the sexual sphere. If a sentence is related in any way to queer identities, the percentage is even higher: depending on the term, hurtful completions appear an average of 13% of times, and up to 87%.
"The phenomenon of offensive completions affects all kinds of identities," Nozza concludes, "but in the case of non-queer identities insults are mostly generic, for queer identities they are, in most cases, about the sexual sphere."