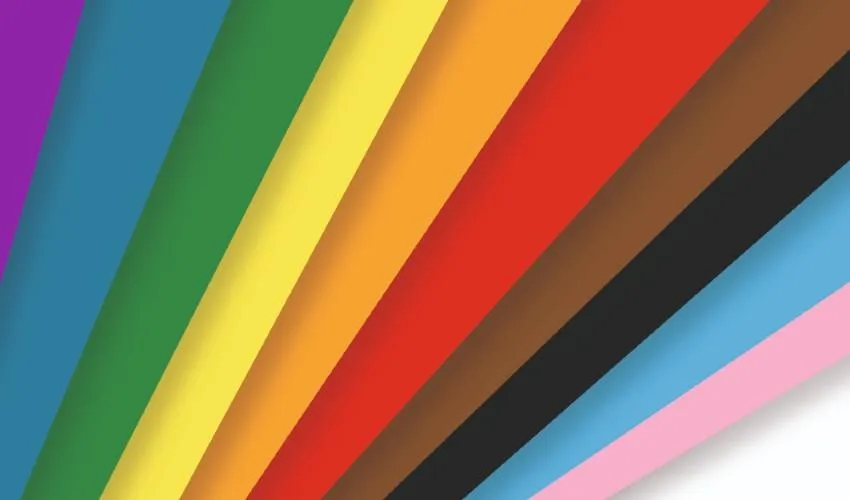
Pride: STEM Disciplines Fight Algorithmic Bias
In its fight against discrimination, the LGBTQIA+ community finds an unexpected ally: the STEM (Science, Technology, Engineering and Mathematics) disciplines. Indeed, it is not only people who discriminate today, but also artificial intelligences, through the bias of algorithms that govern their operation, often beyond the will of their creators.
In one example, on Equal Pay Day 2019, the Berlin subway operator decided to offer a 21% discount (which equals the average gender pay gap) to women, entrusting the choice to a facial recognition system, which is ill-suited to distinguish transgender people. Another concerns apps linked to wearable devices that monitor exercise and wellness struggle to adapt to non-binary individuals.
"The roots of algorithmic bias can be technically very complex," says Luca Trevisan, a Professor of Bocconi's newly established Department of Computing Sciences, "and it is therefore difficult to discuss it apart from the technical aspects. STEM disciplines can model discrimination mathematically and can identify problems and possible solutions." To address the issue, Professor Trevisan, in collaboration with EDGE, has organized the meeting "Fairness in Artificial Intelligence - A mini-workshop on bias and discrimination in AI" (Bocconi University, June 27), which will be attended by scholars from Harvard, Stanford and Bocconi, among others, and will also touch on the legal implications of the topic. The meeting is the first initiative of EDGE's "A+I: Algorithms + Inclusive" project.
In general, all machine learning systems, which are trained to make decisions based on a set of past decisions, risk perpetuating implicit bias and may work less than optimally with minorities. This is the case, for example, with machine translation technologies, which, as Dirk Hovy explains in the article below, risk making only the speech patterns of middle-aged straight males seem legitimate and struggle to understand the use of modern pronouns, which are being introduced to denote non-binary sexual identities.
It can be demonstrated that even popular language models used, for example, by Google to correctly interpret user searches, incorporate subtle and insidious forms of bias to the detriment of the LGBTQIA+ community and women.
If we rely on an artificial intelligence system to pre-select the CVs of candidates to a certain job, we risk perpetuating the gender bias that may have led, in the past, to hiring only males for top management positions. The immediate ("but crude," Trevisan warns) solution might be to purge CVs of any reference to the gender of the candidate. "Gender, however, can be correlated with a lot of other information such as the university course attended or the adjectives chosen to describe themselves, and a machine learning system would, in all likelihood, continue to pre-select mostly men."
The poor performance of facial recognition systems when dealing with minorities can be traced to one technical aspect, called regularization. "In setting up a machine learning system, you always try to achieve a certain balance between model complexity and accuracy of prediction," Prof. Trevisan explains. "It is a sort of application of Occam's Razor principle, which prefers a simpler 'explanation' of the data (a model, or a decision rule) to a more complicated one, even if the simpler one is slightly less accurate. In this way, to return to our example, the risk is to settle for a facial recognition system that is effective for almost all white males but for very few members of all minorities underrepresented in the set used to train the system."
Finally, machine learning models are extremely complex and can include millions, if not billions, of parameters. When a model makes a decision, a prediction or classification, it does not provide an explanation for it. "Getting 'explainable' predictions and diagnosing what discriminatory decisions are derived from requires very specialized STEM skills," says Trevisan.
Even the best intentions can, in fact, have paradoxical effects. Algorithms that try to detect online hate speech, for example, often end up classifying the words that designate the targets of attacks ("woman" in the case of misogynistic attacks, or "gay") as offensive. Fortunately, some solutions are possible, as Debora Nozza points out in the article below.
Examples of algorithmic bias are still relatively uncommon, and the risk is to underestimate their significance. "But given the rate at which technology is progressing, examples will soon appear on a daily basis," Trevisan cautions. "The situation reminds me of privacy issues in the 1990s. For a while they were dealt with only by scholars, but within a few years the issue exploded. Italy was at the forefront in that case, thanks to scholars like Stefano Rodotà. We can hope that the same can apply to algorithmic bias."
As in the case of privacy, constitutionalist Oreste Pollicino explains in the piece above, European law aims to strengthen users' position through the introduction of mandatory procedures for platforms, and especially for the so-called "very large platforms" that, by collecting more data, have also greater opportunities to profile users.