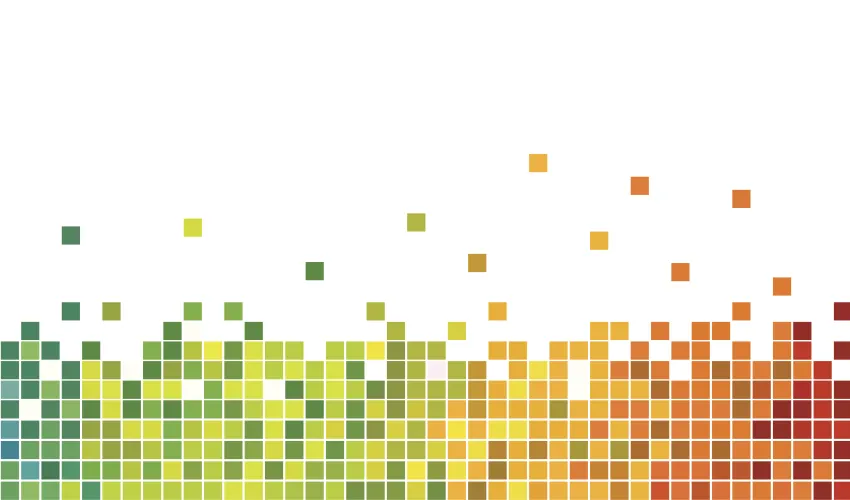
The Concrete Impact of Artificial Intelligence
By now, everybody should know that the recent progress of Artificial Intelligence (AI) is producing a dramatic impact on many sectors of our societies. AI has indeed obtained spectacular results that would have been considered impossible fifteen years ago. It can now process images automatically, identify faces, segment images and provide a semantic description of their content, opening the way to self-driving cars or trucks. Over the last decade, voice recognition and automatic translation have progressed more than in the previous five ones. Algorithms are competing with the best professionals at analyzing skin cancer symptoms or at detecting specific anomalies in radiology.
Recent AI algorithms have shown surprising ability to detect hidden correlations as well as relevant variables and patterns in massive sets of complex data. In some sense, they provide an efficient way to extend the basic tools of statistics to "large dimensional" spaces where one wants to identify many control variables and their relevance, using large data sets. Hence their growing importance in all types of profiling tasks, ranging from the recommendation systems for customers, to personalized medicine. For instance, applying AI to individual data that is being generated in massive developments of "omics" data in biology (analysis of the genome, of the transcriptome -the expression of genes-, of the proteins and their interactions), is prompting a major new step in the long quest of medicine towards more individualized diagnosis and treatments.
Indeed, the remarkable leap of performance achieved by AI in the last decade is well documented in some benchmark scientific problems. The most remarkable of them seems to be protein folding. Finding the shape of a protein (and therefore the organization of its active sites which governs its interactions with other molecules) has been one of the major scientific problems of the last few decades. And just last year, an AI algorithm, AlphaFold, won a world protein-folding challenge with such a decisive margin that many consider it as a true revolution in biology.
Although AI research started 70 years ago, its breakthrough in all these applications -and many more- only began in 2012, with the use of "deep networks" for image classification. The basic architecture of these networks is surprisingly simple. Taking inspiration from the organization of the brain, they consist of successive layers of "artificial neurons", logical units that receive information from neurons in the previous layer, perform a simple computation and, in turn, send a few bits of information to the next layer. If, in the first layer, for instance, the network is presented with the image of a skin lesion, in the last one, it should tell you how likely this lesion is a cancer.
In fact, a modern "deep" network with hundreds of layers, analyzing a large image, can contain hundreds of millions of adaptable parameters ruling these elementary computations. To determine these many parameters, one uses machine learning: one trains the network by presenting a large "training set" of examples for which the results are known, and the parameters are adapted until the network performs the desired task on this training set. The generalization performance of the obtained machine is then tested on new data that the machine has not seen yet.
The paradigm of layered neural networks has been around for over 50 years. So far, it had not been successful on real-size practical applications. Its revival is mainly due to the increase in computing power, to the availability of very large -and labeled- data sets for training, and to many pre-processing and training tricks developed in the 2000's.
In contrast, the scientific understanding of deep networks lags way behind. First, the learning process, in which one finds hundreds of millions of parameters by fitting data remains poorly understood. Even worse, successful fitting with that many parameters should lead to "overfitting", namely poor generalizations on new data. At a more abstract level, what remains a fascinating scientific challenge is understanding how the information, stored collectively by the neurons inside each layer, becomes more global and more abstract when one goes deeper into the layers. Indeed, well-trained deep networks perform amazing tasks, and one knows every single detail of their artificial neurons. In a sense, they become neurobiologists' dreams, "brains" in which one knows all neurons and the efficacy of all synapses... Yet, despite this detailed knowledge, we do not understand how the final task emerges from the collective behavior of many neurons; furthermore, we cannot explain it in simple terms. Solving this challenge will need inputs from statistics, information theory, computer science, theoretical physics, probability theory, computational neurosciences among others...
On top of these scientific questions, the fast AI technological revolution challenges the organization of our societies and poses many ethical questions which call for new regulations. However, as far as global "intelligence" is concerned, these machines have not made much progress. They can achieve specific tasks, characterized by simple answers, yet they are far from building a representation of the world, or from any kind of creative reasoning. In science, deep networks and new data-science algorithms have become extremely useful additions to our toolbox, comparable to the appearance of numerical simulations a few decades ago. However, these machines cannot replace modeling, i.e. building a concise, workable and predictive representation of the world. Contrary to some bold claims, they will not kill the scientific methods that we have been using for five centuries. Rather, they will help it.