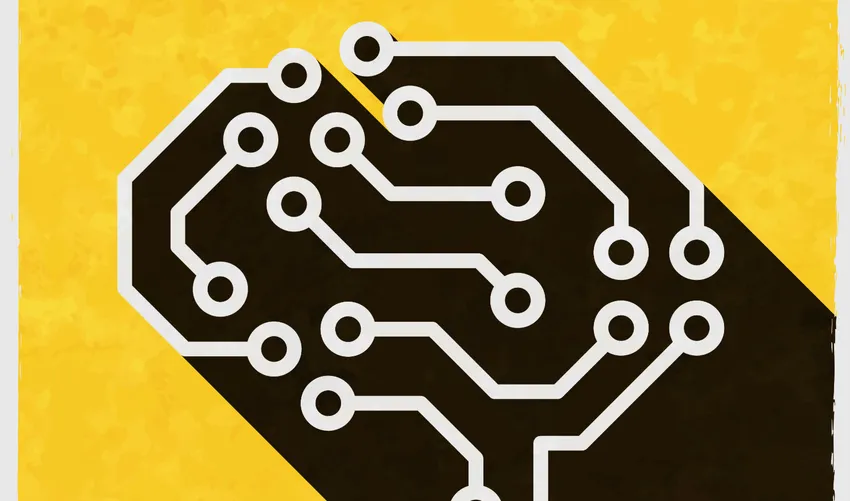
Recycled, augmented but not yet human
Although Artificial Intelligence might seem a recent trend, this idea was in fact introduced in the late 1950s. Since then, public perception, research activity and funding has undergone spectacular ups and downs, including the famous AI winters. Considering the most successful AI achievements of the last decade, albeit fascinating, the original "aspiration of realizing in software and hardware an entity possessing human-level intelligence"[1] has been progressively reframed into the more tangible design of machines that can perform, with impressive accuracy, several human tasks such as text and image generation, object recognition and motion, without necessarily requiring a full understanding of the source mechanisms that make these tasks possible in humans. This is accomplished by leveraging massive amount of data produced and labeled by human intelligence for training Statistical Machine Learning algorithms to succeed in these tasks.
Recalling the recent AI achievements (e.g., ChatGPT[2]), the beauty of this result is that a secondary output of the source mechanisms underlying human intelligence, i.e., human-generated data, is often enough, if combined with advanced algorithms, to create an intelligence capable of mimicking the tasks underlying the creation of the original data. As such, this form of intelligence could be understood more precisely if referred to as "recycled intelligence"[3]. This definition is even more explicative if we consider that not only the input data are a product of human intelligence, but also the powerful computer architectures currently used to process these data have recycled the invaluable and partially-unacknowledged contributions of the early 20th century human computers[4]. Namely talented humans that, in the absence of powerful computing infrastructures, were asked to perform complex computer-type calculations. A sort of reverse-AI requiring humans to mimic computers, rather than the opposite, which however set the stage for the rise of modern computing technologies.
Viewing the current forms of AI in terms of recycled intelligence helps to contextualize its achievements and clarify its differences from another fundamental perspective of learning from data, namely what can be defined as "augmented intelligence". Albeit sharing a common emphasis on data, models and algorithms, this perspective prioritizes the creation of new knowledge about the generative processes and causes of the phenomena at a population level from the analysis of multiple data sources. Hence, it augments human intelligence by coordinating and combining different disciplines and field expertise to answer challenging scientific questions that a single human or a single community would not be able to address autonomously. This perspective, which is a distinctive feature of Data Science, has at its core a form of hypothetical reasoning to design generative models that can produce knowledge, even in the absence of a large amount of training data.
A timely example is Network Science, which is the discipline that analyzes complex connectivity structures among a set of entities. There, the sample size is often one, since only a single network is typically observed. To cope with this problem, structured and generative statistical models, incorporating also prior information from field experts are required. Working in this direction with colleagues from the BayesLab and PhD students from the Bocconi program in Statistics and Computer Science, we have been able to generate new knowledge on the structure and function of brain networks, and on yet-undiscovered pyramidal architectures in criminal networks. In the context of criminal networks, we are now exploring new intersections among factor models from Social Sciences, phylogenetic trees from Biology, field-expert knowledge in Criminology and advanced inference methods from Statistics to further obtain unprecedented reconstructions of organized crime evolutionary histories rooted in the complex interactions among criminals.
Recycled and augmented intelligence have, and will, progressively reshape our way of intending, producing and analyzing data to support complex decision-making tasks. Their synthesis might be the solution to achieve the very original AI aspiration. [1] Jordan, Harvard Data Science Review 2019 (https://hdsr.mitpress.mit.edu/pub/wot7mkc1/release/10)[2] https://openai.com/blog/chatgpt/[3] Donoho, Harvard Data Science Review 2019 (https://hdsr.mitpress.mit.edu/pub/rim3pvdw/release/7)[4] https://www.history.com/news/human-computers-women-at-nasa