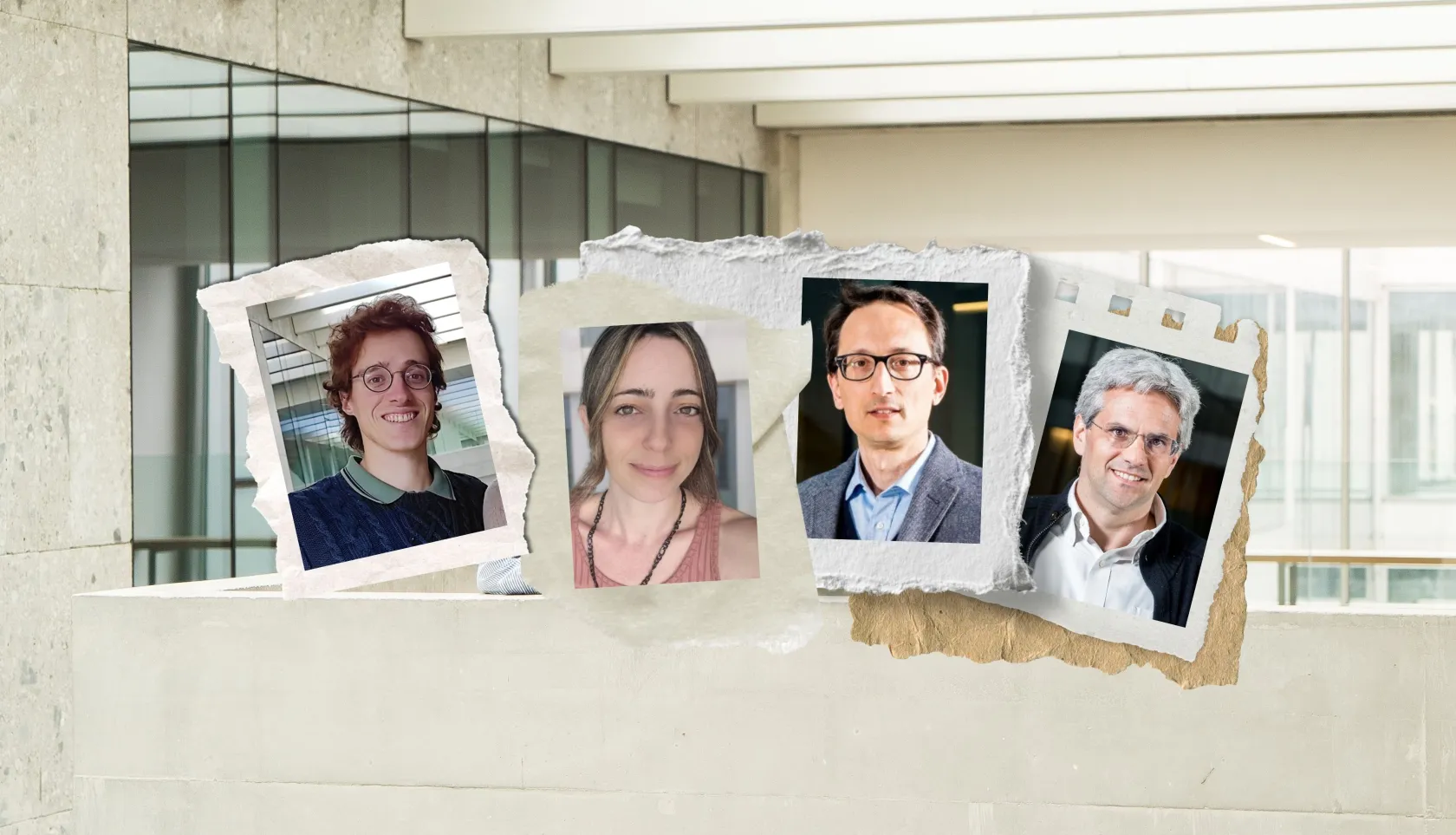
A New Model to Manage Every Correlation
In Bayesian statistics, i.e. the approach that enables the updating of knowledge about a phenomenon using probability measures, modeling the dependence between heterogeneous data is crucial. In fact, developing a model allows you to integrate different sources of data to improve the results of the analysis, avoiding conclusions being based only on a single sample. However, modeling this dependency can sometimes be very complicated. This happens especially in the case of complex models, as in the case of nonparametric Bayesian models. In fact, existing models are limited to modeling positive correlations between data from different sources: an appropriate hypothesis only when data collected from different sources tend to vary in the same direction.
Filippo Ascolani, Beatrice Franzolini, Antonio Lijoi, and Igor Prünster, researchers and professors at the Bocconi Institute for Data Science and Analytics (BIDSA), managed to overcome this limit, introducing a new model capable of managing any type of correlation in their paper “Nonparametric priors with full-range borrowing of information”. In detail, the study outlined a CRM model (Completely Random Measures) with Full-range Borrowing of Information (n-FuRBI). The model combines the flexibility of random series construction with the analytical tractability of CRMs. This is achieved thanks to a new concept, called hyper-tie, and represents a direct and simple measure of dependency.
The key idea of the new model by Ascolani and colleagues consists in the fact that the correlations between data collected from different sources are determined by the links between the latent parameters that generate them. In existing nonparametric models, the parameters corresponding to two observations collected from two different sources, which can coincide or be independent. In this new model, they can be dependent even without necessarily coinciding. This new latent structure allows them to obtain more flexible models, which also allow negative correlation between different data sources.
The model was tested by researchers on both simulated and real data. In the latter case, it was used to predict stock and bond returns and to group students into clusters based on their results on certain tests. The new model showed superior performance compared to other existing methods, providing more accurate predictions and more precise clustering ability, even in the presence of missing data.
In terms of predictions, the n-FuRBI model offers greater flexibility, being able to incorporate both positive and negative relationships between different sources. This allows more precise estimates to be made even in complex scenarios, where the variables do not behave homogeneously. Finally, n-FuRBI models also allow for a variety of interesting extensions. In fact, such models can be seen as effective building blocks for modeling non-trivial dependency relationships in the case of more complex data analyses.
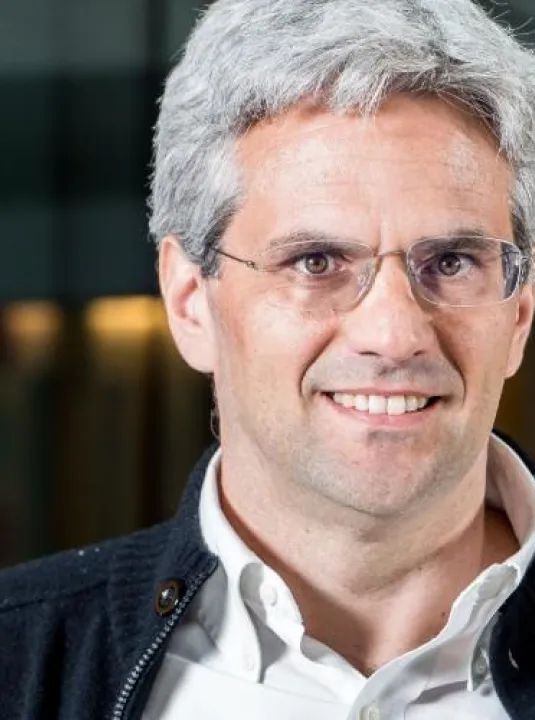
IGOR PRUENSTER
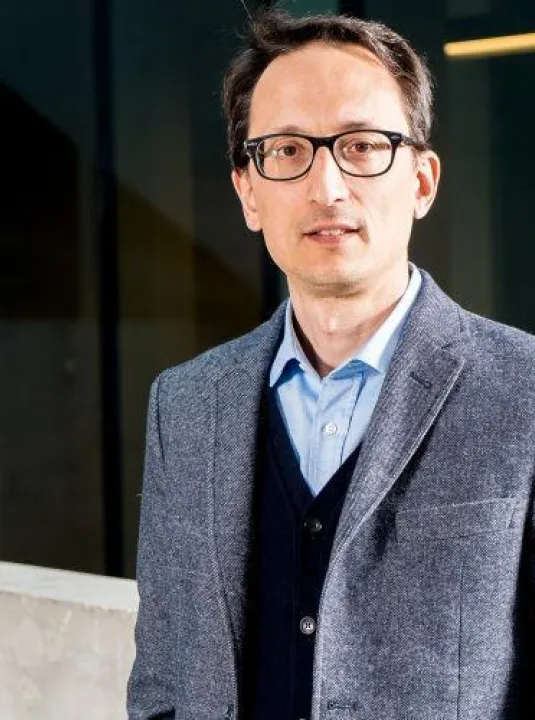