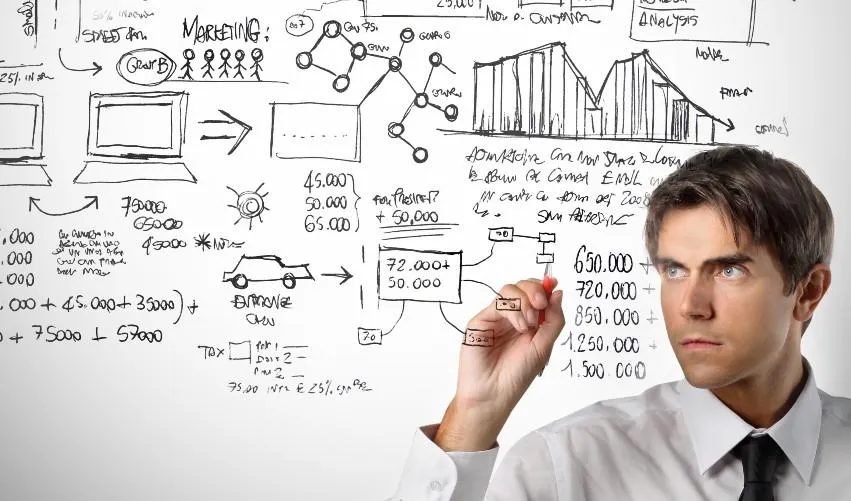
Methods and Models for Deciding with Data
What kind of questions should managers pose to machine learning software? And how can they get the right insights from these tools? These are some of the questions animating two research projects which involve Emanuele Borgonovo, Bocconi Professor at the Department of Decision Sciences. The first is in collaboration with Manel Baucells, (Darden Business School), Elmar Plischke (Clausthal University of Technology), John Barr and Herschel Rabitz (Princeton); and the other is in joint with Jan Rivkin (Harvard), Nicolaj Siggelkow (Wharton), Marco Pangallo (Sant'Anna) and Leonardo Rizzo (University of Vienna).
"There are many models in the literature to make sense of the data, and many methods to explain the models," summarizes Borgonovo. "Both aim to identify which methods really help us understand how models work, how they respond to questions, how they react to stress affecting data inputs, and how it is possible to carry out debugging operations which can validate their results."
Creating data science models that are on a par with best-practice outfits involves a costly investment in terms of time, effort and economic resources for any organization; it is essential that managers exploit the work of AI by gaining greater understanding in the functioning of the algorithms and quickly transform the information received into concrete plans of action. However, the models have exhibited recurring critical vulnerabilities, and understanding how data models relate to external variables is essential for a correct interpretation of the results.
"Unfortunately, it has been shown that different methods applied to the same model and the same data do not provide the same answer," confirms the researcher. "A classic case is that of correlation between the data: often the models do not know how to distinguish whether the dependence observed is functional or statistical, that is, if one varies depending on the other in a cause-effect relationship that therefore always repeats itself, or if the observation is just the result of statistical correlation. It is a fundamental distinction for a manager to make, in order to select the elements on which s/he needs to act to produce change; it is by no means certain that the variables that carry information are those on which it is possible to act. In general, the more the models can be explained, the more they instill confidence, and this is true in business as it is in other fields."
"The second research work cited," Borgonovo concludes, "works on the agent models used to investigate complex phenomena, for example the spread of a pandemic or how people interact with each other faced with factors that are currently important to know and explain."