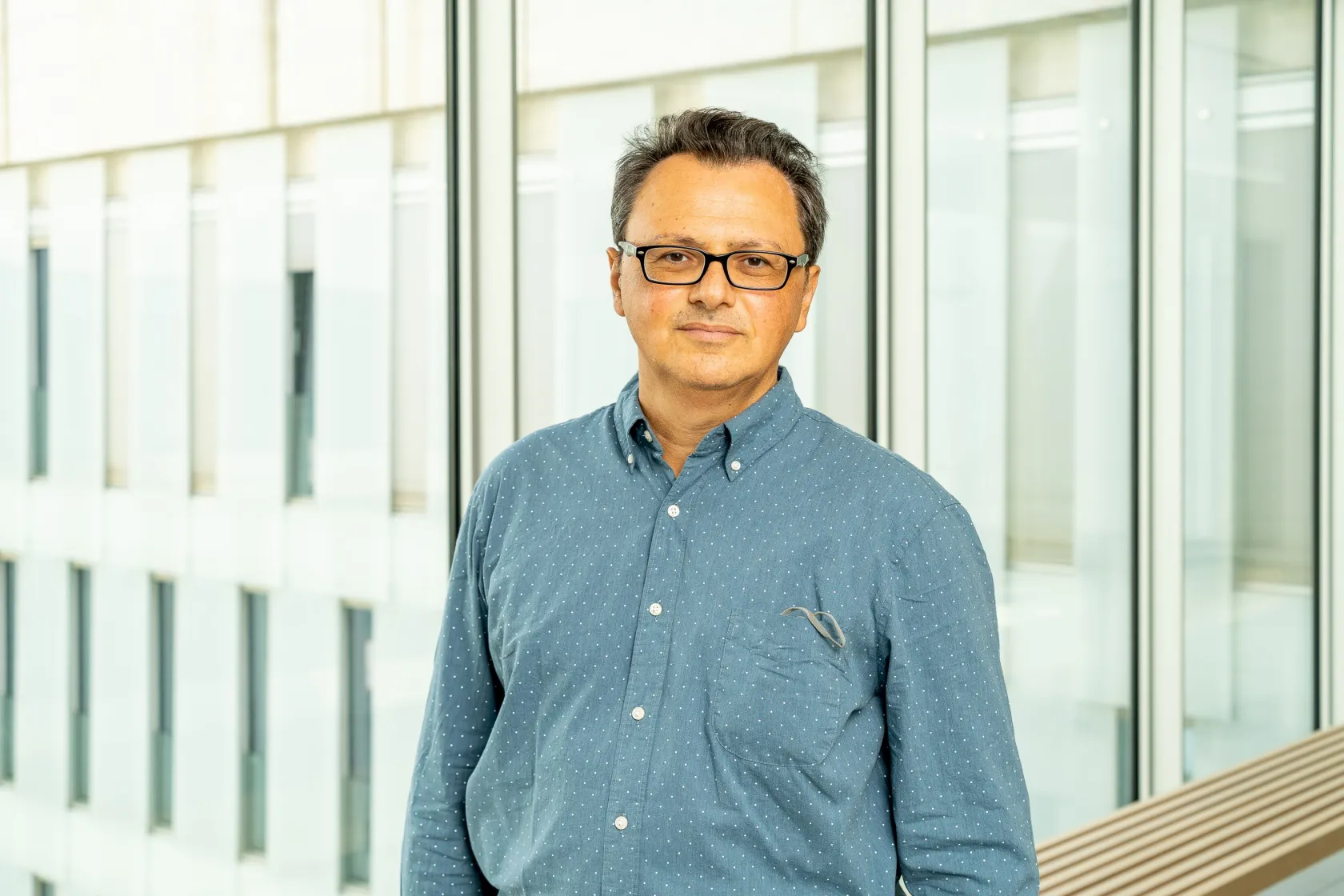
How to improve tax audits
Tax authorities routinely collect enormous datasets on taxpayers and should use them efficiently to audit taxpayers. Are there margins to improve the efficiency of tax auditing? Judges in the U.S. and other countries evaluate hundreds of thousands of defendants for jail or release decisions before trials. These decisions are often left at the discretion of local judges. Can machine learning (ML) help them keep consistent criteria and avoid mistakes and bias?
It may be tempting to see ML problems just as engineering challenges for computer scientists, and we should obviously not underestimate the difficulties associated with designing efficient algorithms. But in many applications, the technical design is the least of the problems. The real complications are in interpreting the results and translating them into appropriate policy recommendations.
Consider the case of a tax authority aiming to design an auditing plan. To be effective, the plan needs to predict the identity of likely tax evaders, a task for which ML algorithms are ideal. In short, an algorithm would use historical data to select the variables that best predict evasion and combine them in a score that could be used for the choice. If this is what the authority does, however, we have a big problem. This procedure would use only outcomes of files that have been endogenously selected for treatment by the authority in previous periods. Any potential bias in the selection process would be inherited by the data. If we can't control for all variables used to drive the selection, the result may be biased decisions, even (and indeed especially) if the algorithm is efficient. For example, imagine that the tax authority selects audits relying also on variables unobserved by the algorithm that are good markers of compliance (perhaps variables that are not stored in official datasets). If we ignore these markers, the algorithm may recommend replacing audited files with unaudited files associated with the unobserved marker. This would lead to an overestimation of the possible improvements generated by ML. Part of the problem here is that we observe the outcomes of (endogenously selected) audits, but we do not observe the outcomes of tax files that have not been audited.
What can be done to address these issues? There is an apparently simple solution: do not use historical data to train the algorithm; instead, use carefully randomized data, as in randomized trials for drug testing. If this were possible, then we would be sure that no bias is present in the training data, and the predictions would be effective. But this solution is often impractical and not what is generally done.
In a recent work, we have proposed a methodology to correct potential biases in the design of tax auditing. We exploit two features of datasets generated by tax authorities: first, only a tiny fraction of files are audited, typically in the single digits; second, files can be audited for up to five years, so there are many unaudited files for which we can assess the true potential since they are eventually audited in later years. These are files that are unintentionally passed over but are then randomly selected for audits at a later stage. Simplifying a bit our approach, we can use them as counterfactuals to evaluate whether we can improve auditing using ML. Specifically, we can use ML to select historically audited files with low potential and replace them with files with good potential, restricting the replacement pool to files for which we eventually see the outcome. This is a conservative policy but one that is arguably immune from the problem that the outcome of the counterfactual is unobserved and may be overestimated. Using data from the Italian Revenue Agency, the analysis suggests that there are indeed large untapped improvements to be gained: replacing the 10% least productive audits with an equal number of taxpayers selected by our trained algorithm raises detected tax evasion by as much as 38%.
Economists have only recently started to think systematically about these issues, which blend ML design with issues more typical of economic research, such as causal identification and optimal policy design and evaluation. There are difficult and sometimes unsolvable tradeoffs in the problems; solutions, like the one described above, may only partially address concerns. Nonetheless, these are promising topics of research because even marginal improvements may be pivotal, and economics has a lot to contribute.