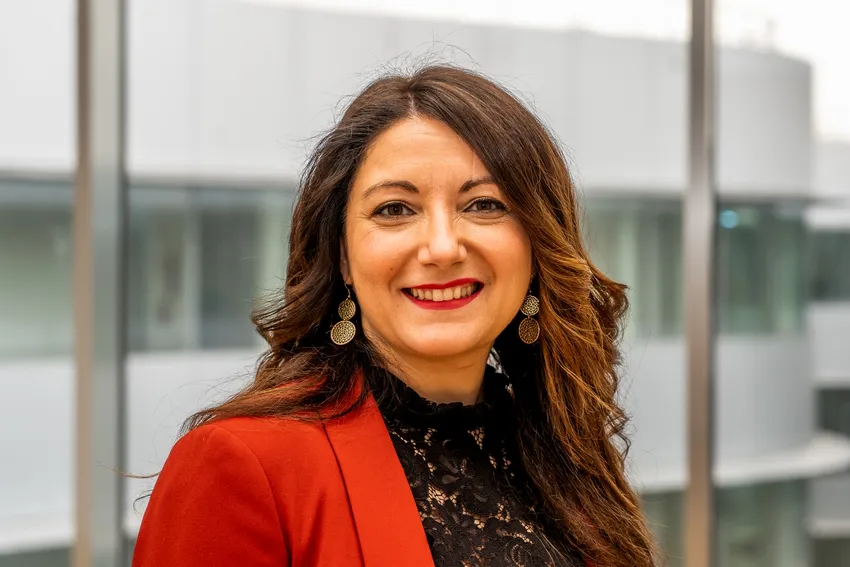
The future lies in Population Health Management
That population is getting increasingly older and the number of people suffering from chronic conditions and multi-morbidities is increasing comes as no surprise. For health care providers, it becomes crucial to sort patients by clusters of needs and risk levels, in order to design targeted intervention according to these needs.
Great potential for personalization and targeted intervention comes from Population Health Management (PHM) approaches and analyzing huge amount of patient-level data, which maps patients' journeys across multiple sectors, and health providers, such as primary care, community care, hospital care, mental health and social care. PHM is a proactive approach to managing the health and well-being of a population that employs analytical tools (e.g. segmentation and risk stratification) to identify the patients, stratify them into groups of people with similar characteristics (for example fragile people with multiple complex health needs or healthy people) and to design targeted pathways and personalized services. PHM also enables effective prioritization, with a focus on identifying the patients at higher risk of developing health conditions or complications and define proactive models of care aimed at reducing hospitalization and death rates.
There are several experiences of application of PHM approaches. For example, several regions in Italy are studying and testing algorithms and models to support the identification of patients, the segmentation, and the selection of these patients for specific care programs. In Veneto region, the ACG system is used to stratify the population in sub-groups with similar needs; in Tuscany region, an ad-hoc algorithm based on resource consumptions is used to identify complex patients, starting from administrative data; in Emilia-Romagna region, the risk-ER logistic regression model is applied to predict risk of hospital admissions or death at one year. Similar methods are also used in several countries all around the world such as Sweden, Germany, the Netherlands, Canada, the US and the UK.
In spite of numerous conceptual models for PHM having been developed, the algorithms used in many of the above-mentioned experiences are still based on a limited number of variables and administrative data, and have been mainly used to describe the characteristics of populations rather than predict future needs. Indeed, predictive models in the management of health populations are relatively new compared with the area of clinical applications.
Looking at the future, thanks to the growing computational power, machine-learning methods and predictive modelling in population health offer great potential to inform proactive interventions, anticipate future disease burden and assess the impact of health policies and programs. Several studies have been recently conducted in the US, using machine-learning algorithms to predict future healthcare expenditures. Even if the Italian health system is very different from the American one, one question is if similar algorithms used to predict costs in the US could be also applied in Italy to flag patients at risks, shorten hospital stays, reduce unplanned hospitalization, and improve readmission rates. Additionally, future projects in machine learning could potentially incorporate larger datasets and additional non-traditional features, such as eHealth data, social media, and web search patterns. To unlock the potential of predictive models for public health the right combination of data, technology, and human intervention is needed. For the time being what will become reality and what will remain utopia is still a matter of debate.