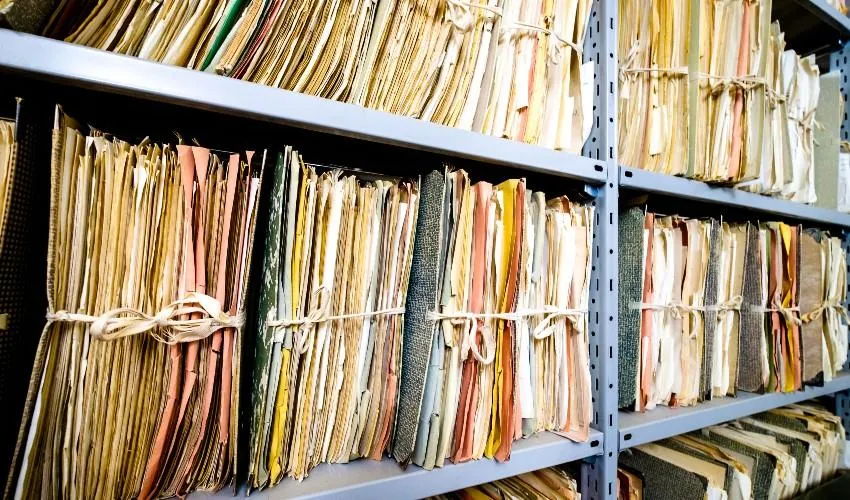
Finding the Perfect Number
If you were tasked with determining what a corpus of 200,000 pages of documents is about, you would face two challenges. The first, of course, is to identify the topics covered in a number of pages difficult to manage manually. The second is to decide how many topics to identify in order to give an answer that is neither reductive (it is unlikely, for example, that three topics would give a minimally exhaustive idea of the topics covered in 200,000 pages), nor unmanageable (with 3,000 topics we would probably be exhaustive, but difficult to interpret).
One of the best solutions to the problem of topic identification is the Latent Dirichlet Allocation (LDA) technique, developed in 2003. Based on it, Francesco Grossetti (Department of Accounting) and Craig Lewis (Vanderbilt University) now propose a solution to the identification of the optimal number of topics through a scientific paper ("A Statistical Approach for Optimal Topic Model Identification", preprint) and OpTop, a package that implements the methodology.
"What we present," Grossetti says, "is a statistical test, which works irrespective of the context and meaning of topics. In technical terms, each topic is an ordered collection of all the words contained in the corpus, whose order represents their importance within a particular topic. It's up to the researcher who uses this tool to interpret the answers, assigning a label to each topic and choosing to merge topics that are very close in meaning, if appropriate."
For his part, Grossetti has already made use of the technique - and the consequent use of interpretive judgment - in a paper on financial disclosure, which identifies the risk factors made explicit by companies in their financial statements.