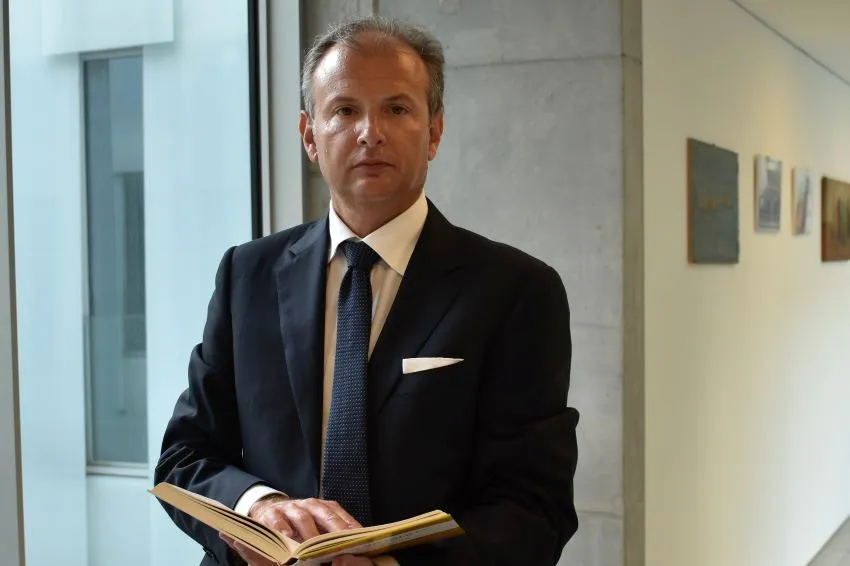
Einstein is the example to follow
The scientific world is debating intensively on how to make artificial intelligence (AI) more transparent. Companies are introducing AI and statistical machine-learning tools at a greater pace in all aspects of their business. While these innovations tend to benefit us to a great extent, scientists, philosophers, and writers raise serious concerns about the threats that an acritical deployment of AI tools poses to mankind. Investigators highlight the tension between automation and augmentation. With automation, we delegate tasks to machines and possibly allow humans to devote time and resources to more creative tasks. With augmentation, we promote cooperation between humans and machines, with machines that make the human intellect more powerful. However, the impact of artificial intelligence can be disruptive: the uncontrolled release of chat GPT has caused unprecedented alarms in schools and educational institutions at all levels, worldwide. Then: should we fear this AI revolution or enthusiastically embrace it? Certainly, if AI remains a set of obscure calculations, then fear and severe criticism opposing an uncontrolled AI diffusion should be the natural reaction.
Already in a 1970 paper about the interaction between managers and numerical models, John C. Little of MIT mentioned that managers refused numerical indications when these were derived from models that remained obscure to the manager. Nowadays, our mentality is much more open to the use of data and of statistical and mathematical models that extract information. Nonetheless, analysts still run the risk of seeing their efforts rejected by stakeholders. There are several famous examples of failures of algorithms, due to macroscopically erroneous forecasts to unfair or discriminatory recommendations. One action urged by the scientific world is to make AI tools as transparent as possible. How can we this goal? First, by avoiding to use of complex models when interpretable models are yielding the same or a similar accuracy for the problem at hand. Here, by interpretable, we mean that an external user has a clear grasp of how a model performs its calculations. The prejudicial view that high accuracy can be obtained in all domains only with complex numerical architectures is increasingly challenged by researchers and professionals. Spinoffs and start-ups devoted to interpretable AI find increasing success in the market. However, much is still to be done to remove the black-box menace and solve the "absence of theory" problem. In a perfect scientific construct, we have a theory, (axioms from first principles from which theorems can be derived) that yields equations (models), and these models exactly describe a phenomenon of interest in the real world. A wonderful example is Einstein's exact calculation of the sun-light bending, computed even before the actual measurements. This setup is rarely (if never) available in AI applications. In this context, we consider data (or measurements) and create a mathematical model that links a set of features (or inputs) to the target (output) of interest.
However, several potential mathematical functions can play the role of the model for a given dataset. Choosing the best model is only the first step. The model must be scrutinized through proper uncertainty quantification, stability, and sensitivity analysis. Borrowing again from John C. Little, a process needs to start to find out what it was about the inputs that made the output come out as they did. This process needs a combination of tools that allow us to understand what features are important in the response of a machine learning model, whether the model behaves by an underlying physical or business intuition (or theory if possible), and, ideally, to perform an X-ray of the model. This process should become an integral part of the release process before algorithms are presented to the public; this is essential to avoid the societal damages of an acritical use of these technologies.