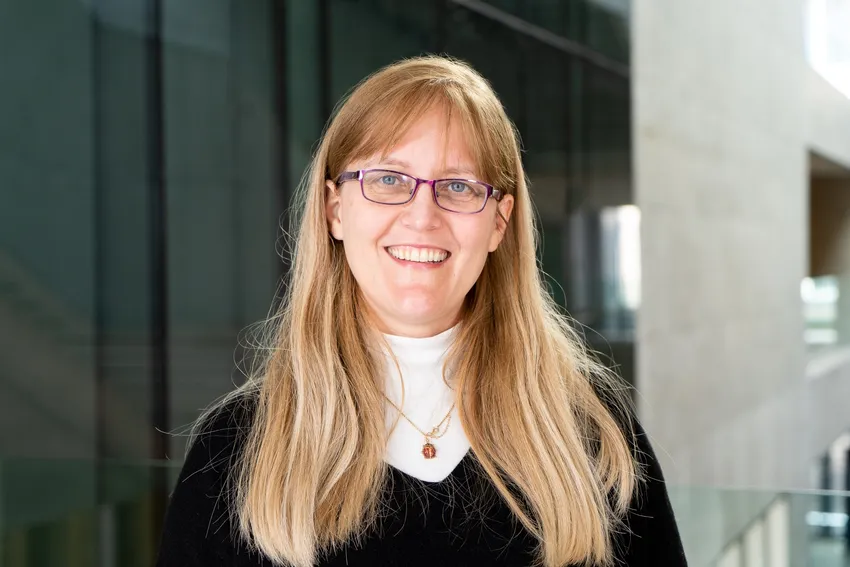
Data that heal
There are great expectations that in the years ahead Artificial Intelligence (AI) will revolutionize medicine. Two key factors are behind this enthusiasm: an unprecedented increase in our ability to acquire patient data, and rapid maturation of methodology to use it. Indeed, AI is already used extensively in this area and is driving important fundamental discoveries and development of new tools. Data production across all health and life science disciplines is growing fast. Collectively, we are acquiring more data than we can make sense of, and thus the power of AI presents us with a tremendous opportunity to provide meaningful and useful analyses of such data. Importantly, not only the speed of data production but also data quality and resolution are rapidly increasing across many disciplines, thus meeting the necessary requirements for AI application.
Genomics - the study of our genes and their function - has arguably seen the greatest recent data explosion, and has caught up other Big Data areas such as astronomy and social media. Indeed, AI is already being applied to analysis of genomics data at all levels, from tackling the challenge of petabyte-scale sequence searches, to identifying genes associated with specific diseases, or using genetic, lifestyle and environment data to predict risk of disease.
By analysing comprehensive biological datasets from healthy controls or people with a specific disease, AI algorithms are likely to also identify promising treatments and guide development of new drugs. One recent AI advance which may lead to faster development of new therapies is AlphaFold, an AI algorithm predicting the structure of many proteins with high accuracy. Resolving and understanding structures can help scientists to understand protein functions, and how such functions may be altered in diseases such as cancer, metabolic or immune diseases. Prior to application of AI to this problem, establishing the structure of proteins was a difficult and time-consuming process that required years of skilled experimental work. Being able to predict such structures with high accuracy will allow us not only to inform development of new treatments for diseases, but also to speed up the drug discovery and development processes.
AI can also help to build models that can predict a patient's response to a particular treatment, based on the omics data obtained from clinical biopsies, before and after treatment, and the individual medical history, leading to more personalized and effective care.
Another area of medicine where AI has shown particular promise is in imaging and diagnosis of disease. Analysis of images such as those acquired by X-ray, CT and MRI scans can improve the detection of abnormalities and identification of clinically significant patterns invisible to the human eye. In this area, deep learning AI algorithms have potential to reveal aspects of the data that would be missed by other methods. This should help radiologists and other healthcare professionals to detect disease at an early stage when treatment is likely to be most effective.
As with any new technology, application of AI to health must meet strict ethical, safety and transparency standards, and this does not come without challenges. Large quantities of high-quality processed data are required for development of useful AI. On one hand, careful primary data curation together with strict preservation of patient privacy and confidentiality are necessary. On the other, multiple levels of data processing, filtering and cleaning come with their own risks, such as making the final data less representative of daily medical practice. Furthermore, appropriate validation and testing of AI models to ensure they are unbiased is critical to avoid perpetuation of existing biases and disparities in healthcare. It follows that there is an urgent need for regulatory frameworks around the implementation of AI, and for adequate training and education of healthcare professionals.
Finally, the use of AI to analyse and interpret healthcare data necessitates parallel evolution of theory, modelling and application. While offering tremendous possibilities, high dimensionality and complexity of this data requires large computing capacities combined with development of new efficient approaches. Last but not least, we need to train a new generation of scientists able to tackle the open questions and work in multidisciplinary teams to develop AI methods that are effective and applied appropriately.