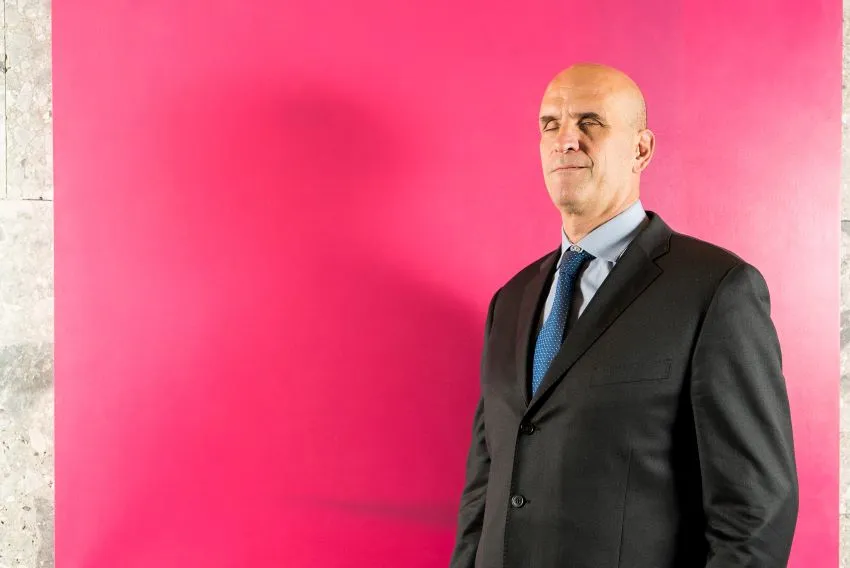
Calculating the risk of conflict
It is clearly very important to have an estimate of conflict risk. In fact, especially in Africa, Asia and Middle East, the country risk evaluated by investors is affected by the risk of conflict. Many civil conflicts, especially in Africa, can be thought of as ethnic conflicts – typically involving the government against an ethnic group. Thus, estimating conflict risk requires as an ingredient to have an estimate of the military strength of each ethnic group. This is due to the fact that, as established in Herrera et al (2022) and Morelli et al (2023), the difference between the military strength and the political power of an ethnic group (relative to the corresponding government) is a crucial trigger of civil conflict.
However, finding measures of military power at the ethnic-group level is extremely challenging. Defining relative military power of an ethnic group as the probability of winning a conflict against the corresponding government, machine learning can be used to obtain such an estimate even for groups that never experienced conflict. In Morelli et al (2023) we use an extended sample of conflicts in Asia and Africa combined with a rich set of ethnic group-level and country-level variables to infer the probability of victory for all potential conflicts between every ethnic (rebel) group and the corresponding government. The advanced learning algorithm chosen has the objective of selecting the relevant predictor variables plus an important cross-validation objective.
We use a stacked ensemble learner, which is a method that combines multiple learning algorithms. Stacking, or Super Learning, is a procedure that aims to find the optimal combination of prediction algorithms. Generally, the cross-validated error of the learner is simply the average error made on each N prediction. The various learning algorithms we use, random forest, gradient boosting machine, and generalized linear models, all have pros and cons, and the stacking procedure improves the risk forecasting performance. To give an idea, previous works on approximations of the probability of winning of an ethnic group were using relative population sizes or nightlight proxies for economic strength. With respect to such previous approximations, we estimate that the machine-learning algorithm improves precision by almost 20 percent. Twenty-percent greater accuracy is quite meaningful in almost any field.
More generally, in social sciences we are often interested in estimating the probability of success of an agent's action as a function of the agent's characteristics, and in the observations available only a fraction of the agents studied did take such actions. Hence using only individual actions and outcomes suffers from a clear selection bias problem. Machine learning allows to estimate the probability of success even for agents who never undertake a given course of action, on the basis of all available data about the set of characteristics of all agents.